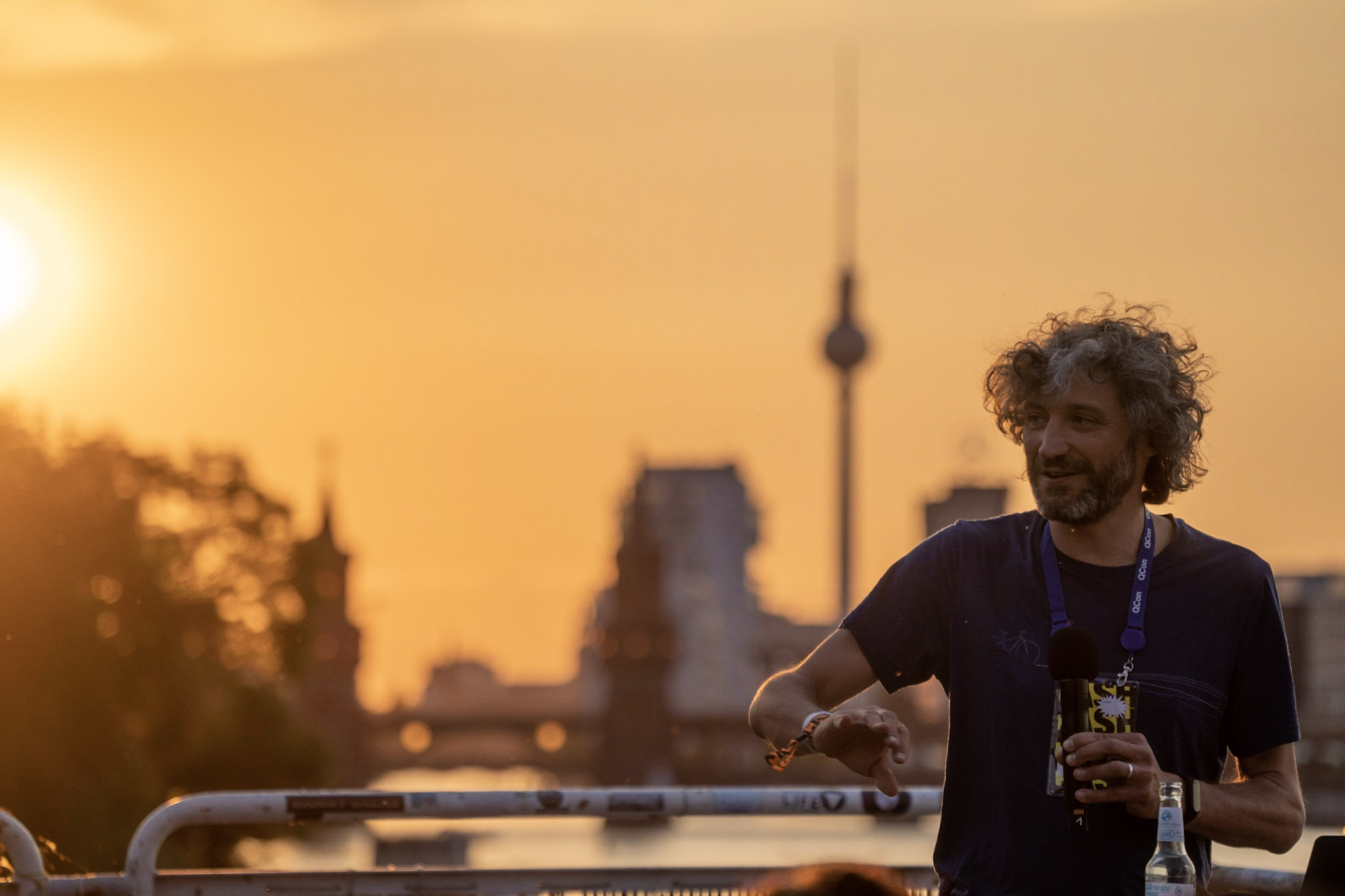
Cloud architect with a strong focus on AWS, data storage, MySQL and cloud cost optimization
AWS Data Hero
Recognized expert on AWS database technologies.
InfoQ Editor
Covering the latest news about cloud computing.
Public Speaker
Speaking and sharing knowledge at international events.
dev.to
AWS is my playground.
-
InfoQ – January 2025
From Apache Hudi 1.0 to the latest controversies in the database world, from AWS Glue 5.0 to data transfer terminals, here are the articles I wrote for InfoQ in January 2025. Read the full article.
-
InfoQ – December 2024
From post-quantum cryptography on AWS, to ‘antirez’ back at Redis, from re:Invent 2024 to a year in review by Cloudflare. A recap of my articles on InfoQ last month. Read the full article.
-
Around the World in 15 Buckets
Today, we’ll embark on a little journey around the world using S3. We’ll copy various objects across AWS regions worldwide, aiming to answer two simple questions: Does the storage class affect the speed? How does the size of the object influence the transfer time? Read the full article.